The AICommunityOWL is a private, independent network of AI enthusiasts. It was founded in 2020 by employees of Fraunhofer IOSB-INA, the OWL University of Applied Sciences (TH OWL), the Centrum Industrial IT (CIIT) and Phoenix Contact. Together, we believe in digital progress through the use of machine learning. We want to create sustainable solutions for the challenges of the future: industry, mobility, smart buildings and smart cities – and above all, for people!
The Machine Learning Reading Group (MLRG) of the AICommunityOWL has the goal to get a better understanding of current trends in machine learning on a technical level. The target audience are researchers and practitioners in the field of machine learning. We read and discuss current papers with a high media impact or prominent positioning (at least orals) of the leading conferences, e.g. NeurIPS, ICML, ICLR, AISTATS, UAI, COLT, KDD, AAAI, CVPR, ACL, or IJCAI. Attendees are expected to have read (or skimmed) the papers that are going to be presented so as not to be thrown off by the notation or problem statement and to be able to participate in informed discussions related to the paper. Suggestions for future papers are encouraged, as are volunteer presenters.
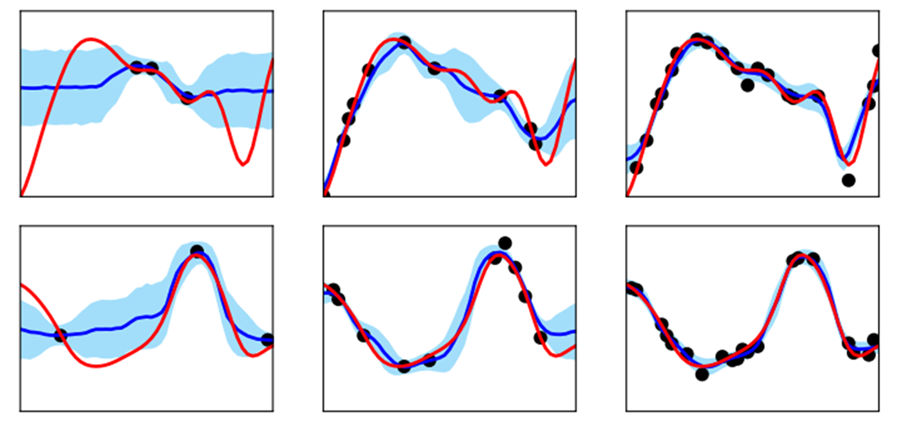
We hold our next online meeting on Tuesday, October 12th, at 16:00 under this link.
Don’t miss the date and save the event to your calendar:
Next Session Title:
Heteroscedastic Temporal Variational Autoencoder For Irregularly Sampled Time Series
https://arxiv.org/abs/2107.11350
Abstract:
Irregularly sampled time series occur in several domains like medical technology, production monitoring, and weather forecasting. They are quite challenging to evaluate with deep learning, since standard models rely on feature complete and continuous data sets. The presented paper introduces an autoencoder based approach for probabilistic interpolation for irregularly sampled time series. The model, called HeTVAE (Heteroscedastic Temporal Variational Autoencoder), includes information about observation sparsity while including uncertainty modeling, to encode probabilistic output interpolation. Thereby the model better reflects variable uncertainty through time in comparison to state-of-the-art methods.
Speakers:
Tom Hammerbacher and Nils Hettig (both Phoenix Contact)
For questions or suggestions of topics, feel free to contact markus.lange-hegermann@th-owl.de
Signup to the MLRG Mailing List to never miss another session!